The Integration of Big Data been associated with assessing risk, calculating premiums, and determining policy terms based on traditional methods such as actuarial data and historical trends. However, in recent years, the emergence of big data analytics has revolutionized the way underwriters assess risk and make decisions. Big data analytics refers to the process of examining vast and varied datasets—often too complex for traditional data processing tools—to uncover patterns, correlations, and insights that can improve decision-making.
The integration of big data analytics in underwriting has the potential to transform the insurance landscape. By leveraging advanced data analytics techniques, insurers can make more accurate, efficient, and personalized risk assessments, improving both underwriting accuracy and customer experience. This article delves into the role of big data analytics in underwriting, examining its benefits, challenges, and implications for the future of the insurance industry.
1. Understanding Underwriting in Insurance
The Integration of Big Data analytics is transforming underwriting, it’s essential to understand the traditional process of underwriting. Underwriting is the process by which insurance companies evaluate the risk associated with an applicant and determine the terms, coverage, and premium for a policy. It involves assessing factors such as the applicant’s age, health, lifestyle, and the condition of the insured property or asset. The goal is to determine how much risk the insurer is taking on and to price the policy accordingly.
Historically, underwriting has relied on a combination of historical data, statistical models, and industry standards. While these methods have been effective, they have limitations, especially in dealing with the complexity and volume of data available today. This is where big data analytics steps in, providing insurers with the tools to analyze a much broader range of data sources and gain deeper insights into risk.
2. The Rise of Big Data in Insurance
Big data refers to the large volume of structured and unstructured data that organizations can now collect, store, and analyze. In the context of underwriting, big data encompasses not only traditional data sources like customer demographics and claims history but also non-traditional data such as social media activity, IoT (Internet of Things) sensor data, GPS information, and even environmental data. The ability to aggregate, process, and analyze these diverse data streams allows underwriters to build a more comprehensive picture of an applicant’s risk profile.
Several factors have contributed to the rise of big data in insurance:
- Technological Advancements: The proliferation of digital devices, sensors, and mobile applications has led to an explosion of available data. IoT devices, for example, can provide real-time data on driving behavior, home security, and even health metrics, all of which are highly relevant to underwriting decisions.
- Cloud Computing: Cloud technology has made it easier and more cost-effective for insurance companies to store, process, and analyze vast amounts of data. This scalability allows insurers to access real-time data and make more informed decisions.
- Advanced Analytics and AI: Machine learning algorithms and artificial intelligence (AI) have significantly improved the ability to analyze and interpret large datasets. These technologies enable insurers to identify patterns and trends that may not be immediately apparent using traditional methods.
3. How Big Data Analytics is Transforming Underwriting
The integration of big data analytics into underwriting is creating a paradigm shift in how insurers assess risk. Below are some key ways in which big data is changing the underwriting process:
A. More Accurate Risk Assessment
Big data enables insurers to move beyond relying on historical data and broad demographic categories to a more personalized, granular understanding of risk. Traditional underwriting processes often use averages or estimates, but big data allows underwriters to assess risk on an individual level. By incorporating real-time data, such as driving patterns from telematics or health information from wearable devices, insurers can make more accurate risk assessments and determine the appropriate premium for each policyholder.
For example, in auto insurance, telematics-based underwriting uses data from connected car devices to track driving habits, including speed, braking patterns, and hours of driving. This data enables insurers to assess how safely a person drives, allowing them to offer personalized rates that reward safe driving behavior. In health insurance, data from fitness trackers can provide real-time information on a person’s physical activity, enabling insurers to adjust premiums based on lifestyle choices and health outcomes.
B. Improved Fraud Detection
Insurance fraud is a significant challenge for the industry, costing billions of dollars annually. Big data analytics can help insurers detect and prevent fraud by identifying unusual patterns and flagging potentially fraudulent claims. By analyzing large datasets from various sources—such as social media, transaction records, and claims history—insurers can detect inconsistencies or red flags that may indicate fraudulent activity.
For example, AI algorithms can be trained to recognize patterns of fraudulent behavior, such as a history of frequent, suspicious claims or claims submitted shortly after purchasing a policy. By incorporating big data analytics into their fraud detection processes, insurers can significantly reduce the occurrence of fraudulent claims and improve the overall efficiency of their underwriting operations.
C. Faster Underwriting Decisions
Big data analytics can greatly speed up the underwriting process by automating data collection, analysis, and decision-making. Traditional underwriting often involves lengthy manual processes, requiring underwriters to review multiple data sources and make subjective decisions based on limited information. In contrast, big data allows for the automation of many steps in the underwriting process, reducing the time it takes to make decisions and improving efficiency.
By utilizing machine learning models, insurers can automatically assess an applicant’s risk profile based on a wide range of data sources, from medical records to environmental data. This automation reduces the need for human intervention and ensures that decisions are based on real-time, accurate data, improving both speed and accuracy.
D. Personalized Products and Pricing
Big data analytics enables insurers to offer more personalized insurance products tailored to the unique needs and behaviors of individual customers. Instead of offering generic policies, insurers can create customized products that provide coverage specific to an individual’s lifestyle, preferences, and risk factors.
For example, insurers can use data from wearable devices to offer personalized health plans that incentivize healthy behaviors. A person who exercises regularly and maintains a healthy weight might receive a lower premium, while someone with a sedentary lifestyle may be offered a higher premium or additional health-related services to encourage healthier habits. Similarly, in home insurance, data from smart home devices (such as security cameras and smoke detectors) can help insurers provide more targeted coverage options and discounts.
4. Challenges in Integrating Big Data Analytics into Underwriting
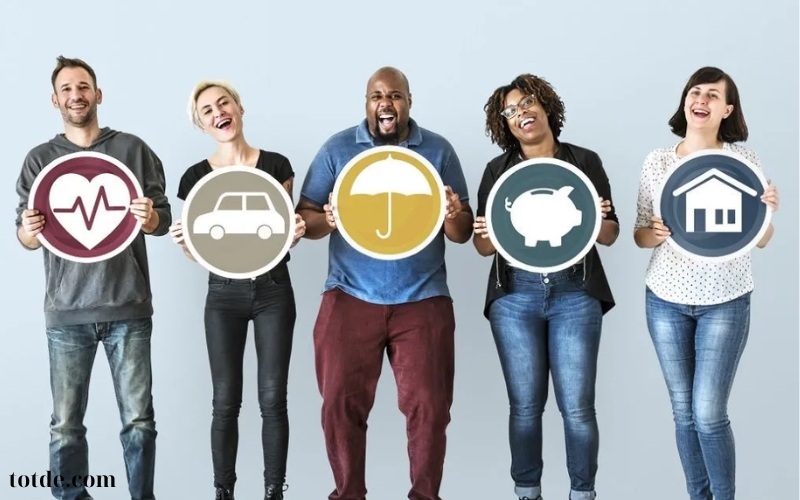
While the integration of big data analytics in underwriting presents numerous benefits, it also comes with several challenges. Insurers must navigate a complex landscape of technological, regulatory, and ethical considerations to ensure that they are leveraging big data effectively and responsibly.
A. Data Privacy and Security
One of the most pressing concerns surrounding big data analytics in underwriting is the issue of data privacy and security. The use of personal data, especially sensitive information such as health records or driving behavior, raises significant privacy concerns. Insurers must comply with strict data protection regulations (such as GDPR in Europe or HIPAA in the U.S.) to ensure that they are handling customer data in a secure and ethical manner.
Furthermore, as insurers aggregate large volumes of data from various sources, they must ensure that this data is protected from breaches and cyber-attacks. Robust cybersecurity measures, along with transparency and consent protocols, are essential to maintaining customer trust and compliance with legal requirements.
B. Data Quality and Standardization
The effectiveness of big data analytics in underwriting depends on the quality and accuracy of the data being collected. In many cases, data comes from a variety of sources, such as social media, IoT devices, and third-party databases, each with different formats and quality standards. Ensuring that this data is accurate, complete, and standardized can be a significant challenge.
Additionally, the integration of new data sources into existing systems requires advanced data governance strategies to ensure consistency and reliability. Insurers must invest in data management tools and processes to ensure that the data they use for underwriting is of the highest quality.
C. Ethical Considerations and Bias
Another challenge is the potential for bias in underwriting algorithms. Machine learning models rely on historical data to make predictions, and if that data reflects biased or discriminatory practices, the models can perpetuate those biases. For example, if an insurer’s data reflects historical discrimination against certain demographics, the resulting algorithms may inadvertently penalize individuals from those groups, leading to unfair pricing or denied coverage.
To address this, insurers must adopt ethical AI practices and ensure that their algorithms are regularly audited for bias and fairness. Implementing transparent decision-making processes and offering explainable AI models can help mitigate the risk of bias in underwriting.
5. The Future of Big Data in Underwriting
The future of big data analytics in underwriting is incredibly promising. As technology continues to evolve, insurers will have access to even more sophisticated tools and data sources, enabling them to make more informed decisions and offer increasingly personalized products. The ongoing development of AI and machine learning will likely lead to more advanced predictive models that can assess risk with even greater accuracy.
Moreover, as the Internet of Things (IoT) continues to expand, insurers will have access to real-time data from a growing number of connected devices. This will allow for even more granular risk assessments and help insurers create dynamic pricing models that can adjust in real-time based on changes in an individual’s behavior or environment.